- 2025년 3월 17일 오전 10:12
- 조회수: 74
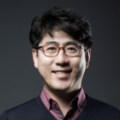
거대 언어 생성 모델인 챗지피티(ChatGPT)와 클로드(Claude) 등의 대표적인 단점으로 흔히 ‘할루시네이션(hallucination)’이 꼽힌다. 이는 생성 모델이 질문에 대해 잘못된 답변을 마치 사실인 것처럼 그럴듯하게 만들어내는 현상을 의미한다. 물론 이런 오류가 문제를 일으킬 수도 있지만, 한정된 학습 정보로 만들어진 생성 모델이 할루시네이션을 보인다는 사실 자체는 오히려 자연스러운 현상이다. 예를 들어, 인간도 비슷한 상황을 겪는다. 가령 옆에 있는 교수에게 질문한다고 해보자. 교수는 잘 아는 분야에 대해서는 정확한 답변을 하겠지만, 익숙하지 않은 주제라면 그냥 "모른다"고 하는 사람도 있을 것이다. 하지만 잘난 체하는 성향이 있는 교수라면, 아는 정보를 조합해 최대한 그럴듯한 답을 내놓을 수도 있다. 그러나 그 분야의 전문가가 듣기에는 그 답변이 터무니없거나 사실과 다를 수 있다. 우리는 종종 "어떻게 저렇게 그럴듯하게 틀린 말을 하지?"라며 놀라곤 한다. 유튜브나 방송에서도 전문가처럼 보이는 사람이 자신감 있게 설명하는 모습을 보고, 실제 전문가들이 "저 사람, 진짜 잘도 속이네"라며 비웃는 경우도 많다. 생성 모델도 마찬가지다. 제한된 정보로 학습되었기 때문에 이런 현상이 나타나는 것이다. 하지만 이는 오히려 인간처럼 사고하고 답변하도록 훈련 받았다는 증거이지, 일부러 아는 내용을 왜곡해 거짓 답변을 하려는 의도가 있는 것은 아니다. 결국, 인간이 아는 범위 내에서 최대한 논리를 맞춰 말하는 것과 비슷한 원리로 작동하는 것이다. 생성 모델은 학습한 지식을 바탕으로 답변을 생성하기 때문에, 충분한 데이터가 없거나 익숙하지 않은 분야에서는 정확한 답을 내놓기 어렵다. 따라서 생성 모델이 할루시네이션을 일으킬 수 있다는 점을 인식하고, 애매한 정보는 신뢰할 수 있는 서적이나 검색을 통해 검증하는 것이 중요하다. 앞으로 인공지능 시대에는 이러한 오류를 판단하고 걸러낼 수 있는 능력이 더욱 중요해질 것이다. 결국, 어중간한 사람들은 퇴출되고, 각 분야의 최고의 전문가들이 인공지능과 협력하며 일하는 시대가 오지 않을까 생각해 본다.
A common drawback of large language generation models, such as ChatGPT and Claude, is “hallucination.” This is when a generative model produces a false answer to a question that is plausible as if it were true. While this error can be problematic, it is actually quite natural for generative models with limited training information to exhibit hallucination. For example, humans experience a similar situation. For example, imagine asking a question to the professor next to you. The professor might give you a precise answer in a subject they know well, but if the subject is unfamiliar, they might just say “I don't know.” However, if the professor has a tendency to be arrogant, he or she might try to cobble together the information he or she knows and come up with the most plausible answer possible. However, to an expert in the field, the answer might sound absurd or untrue. We often wonder, “How can they be so plausibly wrong?” We often watch YouTube videos or broadcasts where someone who seems to be an expert explains things confidently, while real experts laugh and say, “Wow, that guy is really good at fooling people.” The same is true for generative models. The same is true for generative models. They are trained with limited information, which is why they behave the way they do. But this is evidence that they've been trained to think and answer like humans, not that they're intentionally distorting what they know to give false answers. In other words, it works in a similar way to how humans speak, making as much sense as possible within the confines of their knowledge. Because generative models generate answers based on the knowledge they have learned, they are less likely to be accurate in areas where they don't have enough data or are unfamiliar. Therefore, it is important to recognize that generative models can make mistakes, and to verify any ambiguous information with reliable books or searches. In the future of AI, the ability to recognize and filter out these errors will become even more important. Eventually, the mediocre will be weeded out and the best experts in their fields will be working in collaboration with AI.
Seoung Bum Kim. All Rights
Reserved. No part of this document may be cited or reproduced without
permission.