Reinforcement Learning with Human Feedback: Preference-based Reinforcement Learning 3
- 2025년 3월 13일 오후 6:56
- 조회수: 271
INFORMATION
- 2025년 3월 14일
- 오후 12시 ~
온라인 비디오 시청 (YouTube)
발표자:
허종국
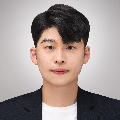
TOPIC
Reinforcement Learning with Human Feedback: Preference-based Reinforcement Learning 3
On-Line Video
OVERVIEW
요약: RLHF란 인간의 피드백만을 가지고 강화학습 에이전트를 학습하는 분야를 가리키며, 도메인 지식을 기반한 구체적인 보상 함수 설계없이 복잡한 태스크를 수행할 수 있음을 시사한다. 금일 세미나에서는 RLHF의 다양한 분야 중 '이진 비교'에 기반한 Preference-based Reinforcement Learning (PbRL)에 대해 소개한다.
지난 1편과 2편에서는 Online PbRL에 대한 다양한 방법론들을 살펴보았다면, 이번 편에서는 Offline PbRL 방법론 3가지에 대해 알아보고자 한다. Preference Transformer는 Transformer 아키텍처를 활용해 선호 데이터에 대한 시계열성과 선호에 기여한 중요 시점을 파악해 더욱 정확한 보상 함수를 추정하는 방법론이다. DPPO와 IPL은 보상 함수 없이 선호 데이터만을 활용하여 에이전트를 학습하는 방법론이다.
참고자료 :
[1] Kim, C., Park, J., Shin, J., Lee, H., Abbeel, P., & Lee, K. Preference Transformer: Modeling Human Preferences using Transformers for RL. In The Eleventh International Conference on Learning Representations.
[2] An, G., Lee, J., Zuo, X., Kosaka, N., Kim, K. M., & Song, H. O. (2023). Direct preference-based policy optimization without reward modeling. Advances in Neural Information Processing Systems, 36, 70247-70266.
[3] Hejna, J., & Sadigh, D. (2023). Inverse preference learning: Preference-based rl without a reward function. Advances in Neural Information Processing Systems, 36, 18806-18827
[4] https://bair.berkeley.edu/blog/2020/06/25/D4RL/
[6] Kostrikov, I., Nair, A., & Levine, S. Offline Reinforcement Learning with Implicit Q-Learning. In International Conference on Learning Representations.
[7] Peng, X. B., Kumar, A., Zhang, G., & Levine, S. (2019). Advantage-weighted regression: Simple and scalable off-policy reinforcement learning. arXiv preprint arXiv:1910.00177.
[8] Garg, D., Hejna, J., Geist, M., & Ermon, S. Extreme Q-Learning: MaxEnt RL without Entropy. In The Eleventh International Conference on Learning Representations.